The new reality of a Natural Language Processing Engineer
How Generative AI has changed the game
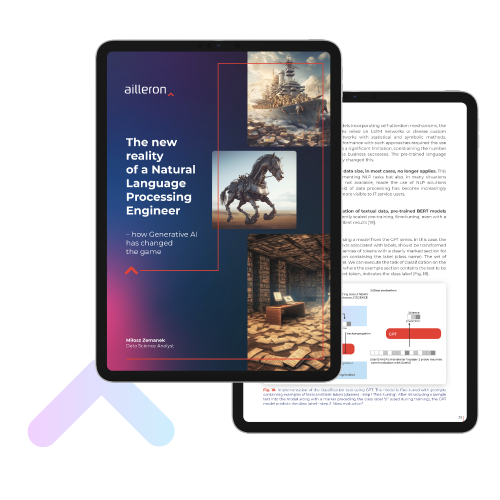
Over the past years, Natural Language Processing (NLP) has undergone a methodological and technological transformation. However, before Generative Pre-trained Transformer (GPT) models revolutionized how we communicate with machines, unlocking unlimited possibilities for businesses, education, and research, NLP had come a long way, dating back to the 1950s. In this eBook, we delve into the history of NLP, focusing on the rise of Large Language Models (LLM). It showcases NLP's varied applications, such as its use in banking, and reflects on its future as an applied engineering discipline.
Generative Pre-trained Transformer (GPT) models have changed the game, introducing unmatched capabilities in generating text that resembles human language. They feature a comprehensive understanding of language with all the cultural and contextual nuances. In our newest eBook, we explore the GPTs' potential and their impact on achieving seamless interactions between humans and machines.
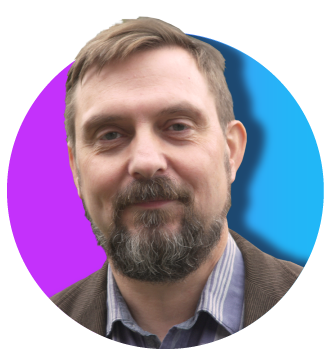
Miłosz Zemanek
Author, Data Science Analyst
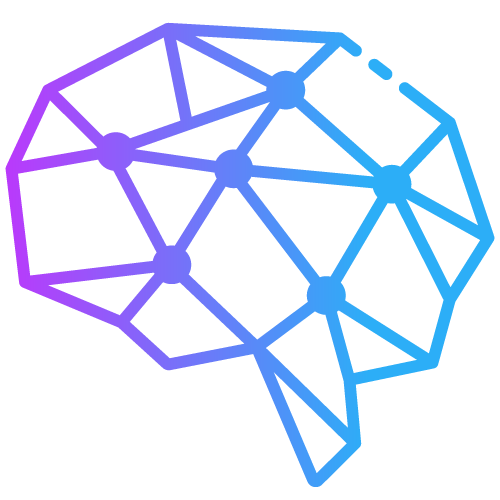
What can you learn from our eBook?
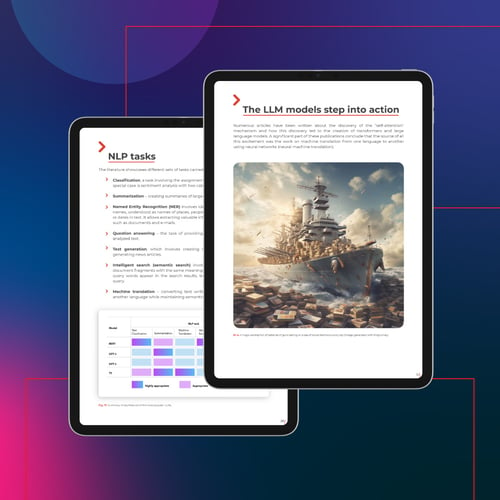
How has Natural Language Processing (NLP) evolved through distinct chronological phases?
How have large language models (LLMs) changed the approach to NLP?
How did LLMs lead to ChatGPT, Gemini, and GitHub Copilot tools?
How has the concept of "self-attention" and transformer architecture impacted the development of LLMs?
For what purposes is NLP commonly employed?
How do the Generative Pre-trained Transformer (GPT) models operate in practice?
GPTs change the way we interact with language-based systems. Pre-learning equips the GPT model with language understanding capabilities, while fine-tuning or alignment enhances its capacity to generate well-adapted responses for tasks like text classification, summarization, or named entity recognition.
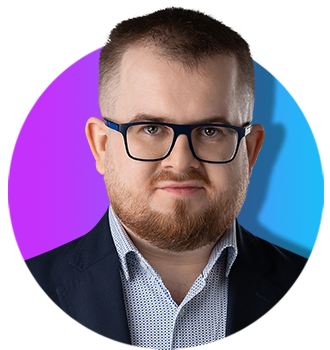
Jakub Porzycki
Machine Learning Team Leader
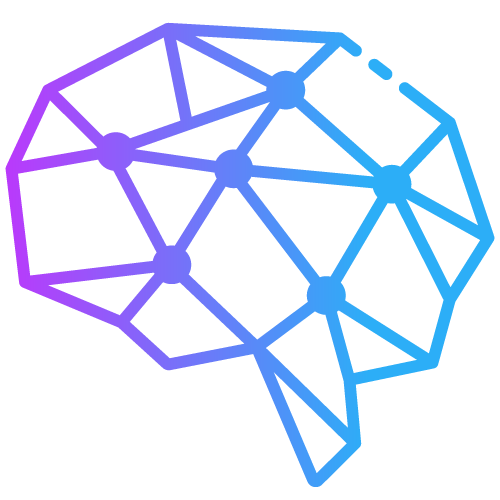