Modern approach to banking customer data representation
How ML deals with hundreds of attributes?
Unstructured data makes up the majority of data in any organization, and using it wisely to uncover business value is one factor that increases market competitiveness.
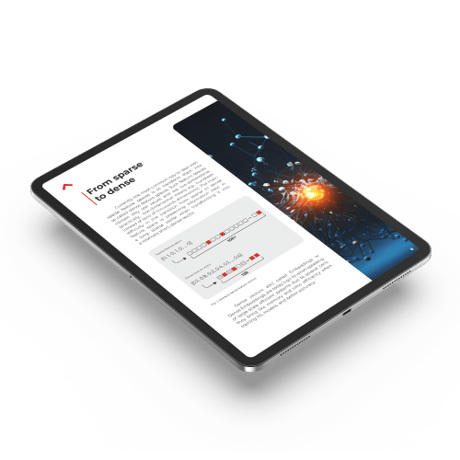
Data context plays a significant role – traditional databases and search algorithms struggle to account for intricate relations within complex datasets. In banking, understanding customer preferences based on past activities and predicting future behavior requires a comprehensive approach.
The response to these challenges lies in machine learning models designed to process sparse and multidimensional customer data and efficiently transform it into context-rich vectors. This eBook addresses this matter by highlighting ML scenarios for finance and banking.
Customer data is one of the most valuable assets that every organization possesses. Efficient differentiation between various types of customers is crucial for accelerating business revenue in multiple domains where personalization is necessary. Using raw data can lead to bias and additional complexity in models. Therefore, our approach to user profile modeling involves processing sparse customer features and timestamped events using machine learning models. This results in compressed representations that are stored in a vector database.
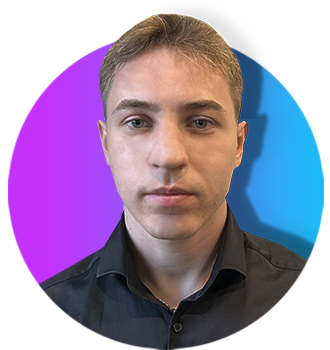
Miłosz Hańczyk
Author, Machine Learning Engineer
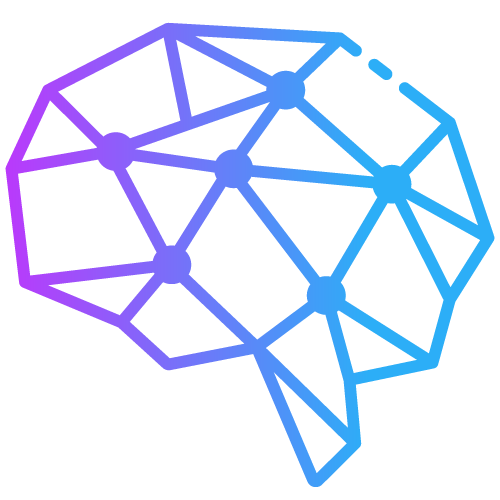
What can you learn from our eBook?
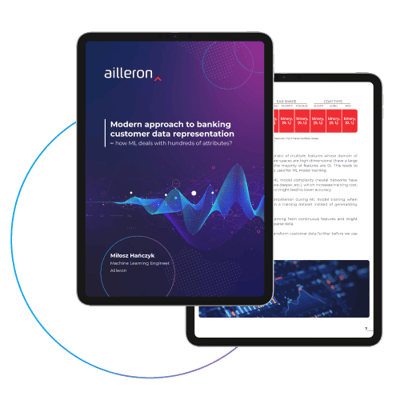
What are the benefits of using machine learning in data processing?
Does proper customer data processing in ML models improve decision-making?
How does user representation influence quality of predictions?
What is the importance of a vector database for user representation storage?
In what scenarios will rich ML-based customer representations prove invaluable?
What are the most well-known strategies for generating embeddings?
Modern Approach to banking customer data representation
- how ML deals with hundreds of attributes?
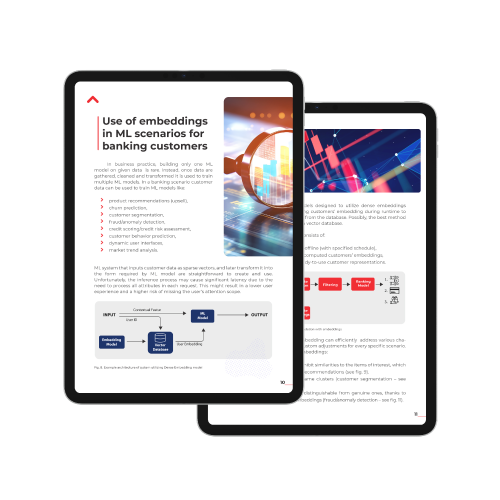
Customer data representation involves simplifying multidimensional data, making it easier to discover patterns and anomalies, and enhancing our understanding of data behavior. It also reduces data complexity, proving valuable across various applications within banking and financial institutions. Ailleron's Machine Learning team primarily focuses on developing industrial machine learning systems for personalized recommendations, behavior prediction, customer segmentation, and other use cases. The following eBook gives an insight into our approach to leveraging the potential of customer data.
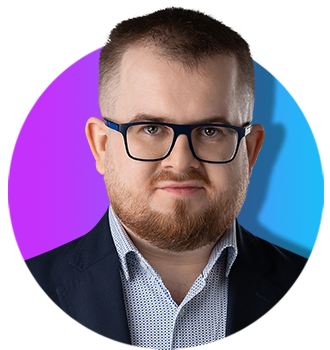
Jakub Porzycki
Team Leader Machine Learning
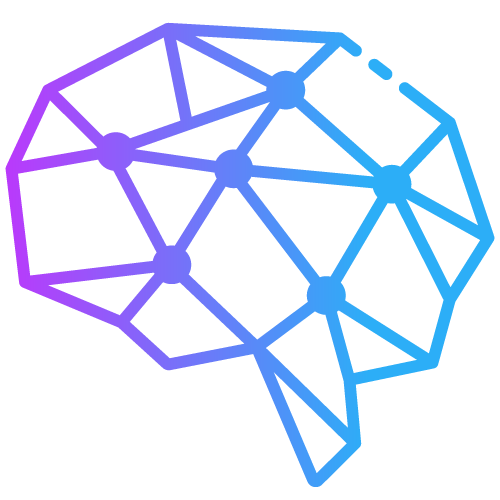