Ageing of machine learning models in finance
Discover data drift and concept drift
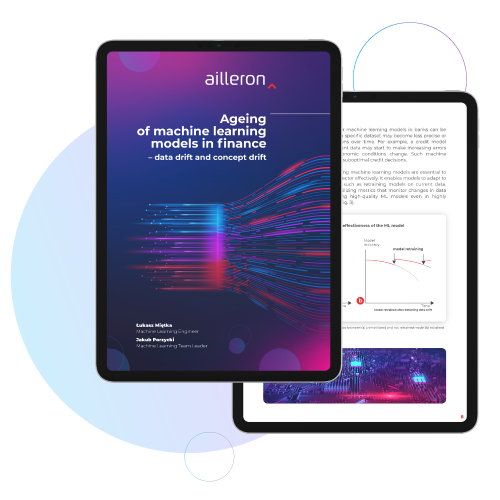
Joint research by MIT, Harvard, the University of Monterrey, and Cambridge revealed that 91% of machine learning (ML) models degrade over time. Two phenomena, data drift, and concept drift, are the main causes of model deterioration. The evolving distribution of production data can impact the model's performance. The results of the Nature scientific journal's study highlight the importance of taking measures to counteract the aging of ML models in production environments.
Data is rarely static in the corporate world – most will change over time, leading to the potential degradation of ML model performance. Therefore, regular monitoring is essential for every production ML model. Adapting to changing data distributions and relationships between features and labels poses a critical challenge. Successfully handling these changes requires continuous monitoring, adjustment, and optimization through prevention strategies, model fine-tuning, and ML-Ops implementation.
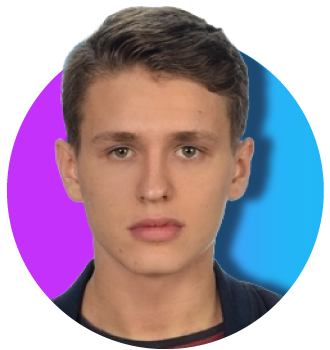
Łukasz Miętka
Author, Machine Learning Engineer
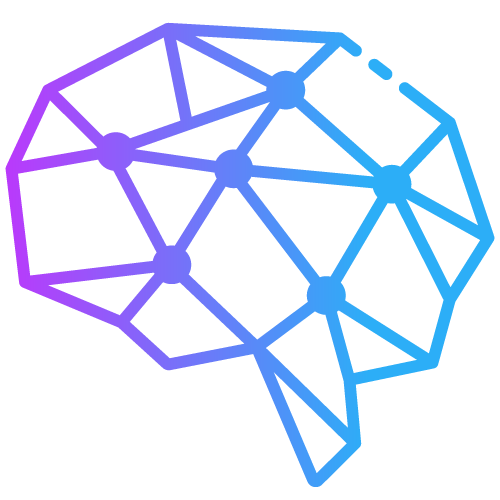
What can you learn from our eBook?
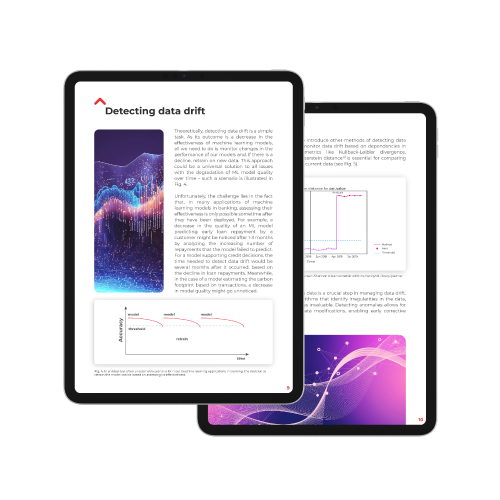
In what areas of banking are ML models applied?
What are the critical issues to focus on when developing ML models for the banking sector?
What is the reason for the deterioration of ML models?
Why should we counteract model performance deterioration in production environments?
In what scenarios will rich ML-based customer representations prove invaluable?
What are data drift and concept drift, and how to prevent them?
What is the future outlook for machine learning applications in banking?
Machine learning models can be applied in various areas of banking and finance, bringing numerous benefits. However, they can also deteriorate over time and become unreliable due to changes in data. This eBook focuses on the impact of data drift and concept drift on ML models' effectiveness. It also delves into ways to mitigate these issues and their significance for the industry.
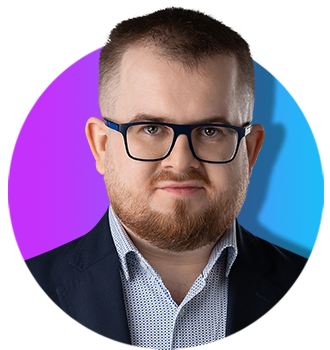
Jakub Porzycki
Co-author, Machine Learning Team Leader
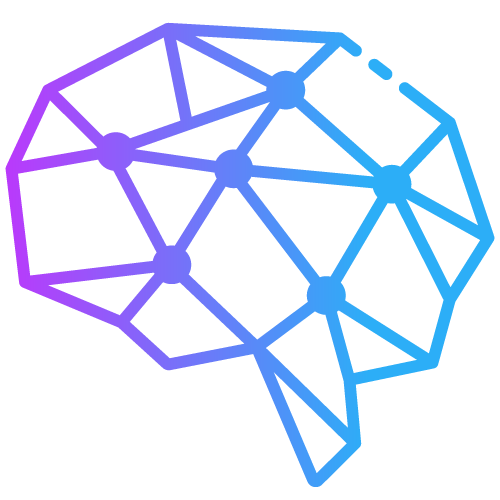